Artificial Intelligence (AI) adoption is accelerating across industries, offering the promise of greater efficiency, automation, and smarter decision-making. From marketing and healthcare to finance and manufacturing, leaders are betting big on AI to transform how work gets done.
But despite those investments, many organizations find themselves disappointed with the results of their AI skills training programs. The core issue? The growing AI skills gap between what employees learn and what they’re able to apply on the job.
Employees may complete courses that explain AI in broad terms, but without clear context or connection to their specific roles, that knowledge rarely translates into action. This disconnect weakens adoption and stalls momentum.
To truly close the AI skills gap, organizations must rethink what effective AI learning looks like. It’s not just about awareness or exposure, it’s about giving people the tools and confidence to use AI in their day-to-day decision-making. That means focusing on real-world applications, job-relevant use cases, and ongoing reinforcement.
It’s time to mind the gap between potential and performance. Without closing that distance, even the most advanced AI strategies will fail to deliver real value.
LEARN MORE: Why Training Without Application is Just Information
Why AI Training Often Fails to Deliver Results
Despite widespread enthusiasm for AI, many training programs fall short in translating knowledge into action. Here are the key reasons why:
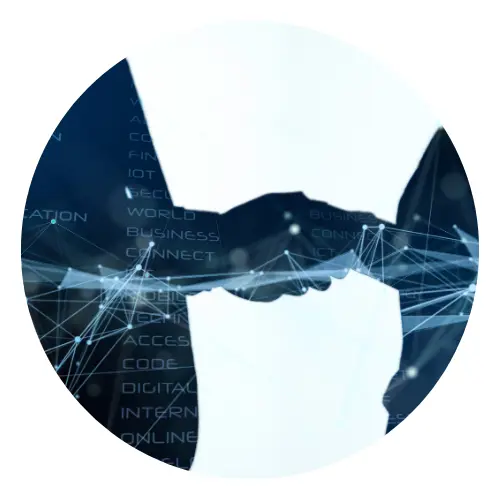
1. Employees Lack Structured Opportunities to Implement AI
Many AI learning programs focus heavily on theoretical concepts but fall short when it comes to practical application. Employees are introduced to the what and why of AI, but rarely get the chance to explore the how, especially in the context of their own roles or business challenges.
This lack of hands-on experience contributes directly to the AI skills gap. Without structured opportunities to experiment, iterate, and apply new knowledge in real-world scenarios, employees struggle to move from learning to execution. They may understand the basics of automation or predictive modeling, but not how those concepts solve actual problems in their day-to-day work.
Effective AI skills training must go beyond slide decks and simulations. It needs to immerse learners in meaningful, job-relevant challenges. Only then can organizations begin to mind the gap between abstract learning and practical, measurable impact.
2. Training Focuses on Theory, Not Real Business Needs
Many AI training programs emphasize technical aspects like machine learning models, data science fundamentals, and AI ethics. While these are important, they often aren’t connected to real business problems. This disconnect is a major contributor to the AI skills gap.
When AI learning doesn’t clearly link concepts to departmental challenges, such as improving customer service, streamlining operations, or enhancing forecasting, employees struggle to see the relevance. As a result, they disengage and underutilize available AI tools.
To truly mind the gap, organizations must design AI skills training that bridges theory and practice. This means showing employees how AI can solve the specific problems they face, and how it fits into their existing workflows. Without that context, even well-trained teams may miss opportunities to innovate and improve performance.
3. Companies Don’t Provide Mentorship or Follow-Up Coaching
Singular AI training sessions are not enough to close the AI skills gap. While initial exposure to generative AI concepts can spark interest, it rarely leads to long-term adoption without continued reinforcement.
According to a report by McKinsey, “48% of employees rank training as the most important factor for gen AI adoption; yet nearly half feel they are receiving moderate or less support.” This gap highlights the difference between offering AI skills training and building true capability.
Employees need more than one-time lessons. They need ongoing support, mentorship, and coaching to gain confidence and competence in applying AI tools to their work. Without that structure, even well-intentioned learners often revert to familiar processes rather than integrate new technologies into their workflows.
To mind the gap, organizations must treat AI learning as a continuous journey, not a one-and-done event. Building a culture of consistent, role-based training and peer support is essential for meaningful, lasting adoption.
The Solution: Hands-On AI Implementation Training
To bridge the AI learning gap, organizations must rethink their approach to AI education. The most effective AI training programs go beyond passive learning and incorporate active implementation strategies. Here’s what works:
1. Real-World AI Application
Organizations that integrate AI skills training with on-the-job projects see significantly higher success rates. When employees can apply what they’re learning to real business problems, AI learning becomes more relevant, sticky, and impactful.
A separate study by McKinsey found that companies using hands-on training combined with real-world case studies achieve 40% higher knowledge retention compared to those relying solely on theoretical instruction. This directly addresses the AI skills gap by giving employees the chance to practice and apply their skills in the flow of work.
Embedding AI training into active projects helps shift AI from something abstract to something essential. Employees not only gain experience, they also begin to see AI as a tool for solving everyday challenges. This approach helps organizations mind the gap between AI potential and real-world performance, while making AI an integral, usable part of the workflow.
MUST READ: Why AI Training is No Longer for IT Teams
2. Cross-Functional AI Collaboration
Encouraging cross-functional collaboration is a powerful way to strengthen AI learning and close the AI skills gap. When teams from different departments, business leaders, data scientists, and frontline employees, work together on AI initiatives, they surface more relevant use cases and build solutions that are easier to adopt.
This kind of collaboration fosters innovation and accelerates adoption. It ensures that AI training isn’t happening in a silo, but is instead grounded in real business needs. By involving stakeholders across the organization, companies create shared ownership and a deeper understanding of how AI can be applied.
To truly mind the gap, organizations must make space for shared problem-solving. When diverse teams engage in AI projects, the learning is richer, the insights are more actionable, and the path to implementation becomes clearer.
3. Ongoing Support and Coaching
Providing employees with continued mentorship, interactive AI workshops, and dedicated office hours ensures they can navigate challenges as they arise. This kind of ongoing support reinforces AI learning and helps close the AI skills gap over time.
Sustained engagement, beyond initial AI training sessions, gives employees a space to ask questions, troubleshoot in real time, and build confidence with new tools. It also encourages a mindset of continuous improvement, which is essential for long-term AI adoption.
To truly mind the gap, organizations must move from isolated training events to a culture of ongoing enablement. When learning is treated as a journey rather than a destination, teams are far more likely to integrate AI into their workflows and drive lasting impact.
Let’s Bridge the AI Learning Gap
AI’s potential is vast, but without hands-on application, AI training alone won’t drive meaningful transformation. Too often, organizations focus on exposure to concepts without giving employees the chance to put them into practice. This is where the AI skills gap continues to widen.
Companies that prioritize structured implementation, real-world projects, and ongoing support create the conditions for lasting change. By grounding AI learning in practical execution, they move from theory to impact, turning knowledge into results.
Now is the time to shift from passive learning to active engagement. When organizations provide the right tools, context, and support, they can mind the gap between aspiration and adoption. The result is a smarter, more confident workforce equipped to lead in an AI-powered future.
At Data Society, we work with organizations to create tailored training programs that equip and prepare employees with the confidence and skills to adopt AI in a responsible and impactful way.
Want to learn more? More insights can be found here.
Frequently Asked Questions
Most AI training programs focus heavily on theory without offering real-world applications. Without structured opportunities for hands-on practice, ongoing coaching, or alignment with business needs, employees struggle to apply what they learn in their day-to-day work.