Part I of this series explored data-sharing opportunities and challenges in government and healthcare, highlighting the possibilities for creating public benefit through efficient data-exchange processes. Part II of this series will move beyond the merits of overcoming the barriers to data sharing to examine institutional mechanisms—both technical and nontechnical—for developing seamless data flow across domains, disciplines, and departments.
A Data-Sharing Culture Shift
Through extensive data-sharing networks, agencies and organizations can unlock tremendous value in the form of improved workflow and discoveries with the potential to drive better healthcare and public service outcomes. A 2013 McKinsey Global Institute analysis estimated that open data from public and private sources could create opportunities and efficiencies across seven sectors totaling $3 to $5 trillion. However, data practitioners across disciplines, entities, and departments must establish effective data-sharing facilities to realize this potential.
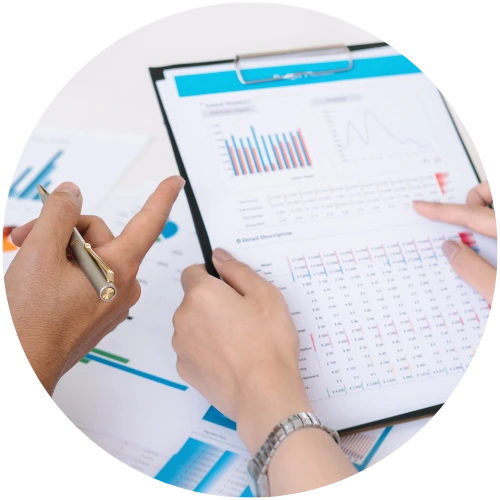
Data sharing on a transformational scale requires a shift in mindset. A Deloitte article urging government leaders to “Reset default to share” argues that the government can offer valuable leadership in increasing data sharing for scientific discovery. The article’s authors note that many fields are historically reluctant to share data. Leaders can help remove this barrier through incentives such as funding for data-sharing initiatives and highlighting the institutional and public benefits of open data exchange.
Increased public awareness of data’s community benefits is also critical for continued progress, especially in encouraging individuals to make their data available. Building trust surrounding who has access to data, how privacy is protected, and how it is used to improve public services and healthcare can help foster a collective sense of ownership and investment in the data-sharing process. Beyond laying the cultural groundwork for expanded data exchange, organizations must develop infrastructures to support secure data flow that cultivates public confidence in data sharing.
Data Management for Healthy Data Flow
As data silos break down and disparate data resources become more broadly available, procedural and technical standards are essential to maintaining data integrity, accessibility, and security. This is where data management is critical.
Data management is an organization’s framework for supporting data governance strategy through skillfully collecting, processing, storing, securing, sharing, and using data. In addition, data management processes oversee data maintenance and timely updates that ensure data points are current, driving real-time analytics that can empower more rapid responses. These processes also facilitate data consistency over the data lifecycle, enabling efficient and secure data sharing by allowing such capabilities as data interoperability.
As a measure of data compatibility, data interoperability is necessary for the integration of diffuse data from diverse sources. Data interoperability requires format and terminology standardization across united data sets, often facilitated by assigning unique identifiers to track data points from different files that pertain to the same entities. Reconciling data pulled from other platforms and often from legacy systems is a challenge that data management professionals undertake to ensure efficient, reliable data exchange and provide a centralized view of what data is available.
Especially germane to government and healthcare organizations, data management also extends to safeguarding privacy to comply with regulatory requirements and engender public trust. Responsible data management can address these concerns through the implementation of protocols for anonymizing personal data, securing data-exchange processes, and ensuring transparency about data’s origins, who has access to it, and how it is used.
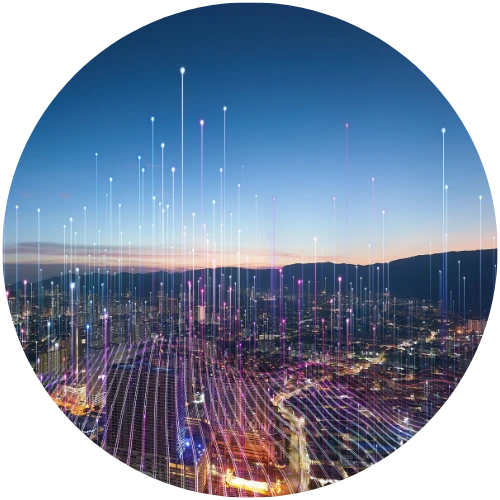
Data Management at Work
Accessing data sharing’s abundant benefits and supporting data management in all phases of the data lifecycle demands various data science skills across roles and departments. Therefore data science training for a range of employees is essential to acquiring the knowledge and tools that drive effective data management. Some organizational functions that are often central to their organizations’ data management ecosystem and can benefit from data science training for themselves and their teams include:
- Chief Data Officer / Chief Data Scientist: An organizational leader serving in this capacity has a particular interest in upskilling teams to perform internal analytics effectively. Workforce skills essential to supporting these data professionals’ goals include foundational business applications for decision-makers and coursework in communicating data-driven insights, such as data science for executives, fundamentals of data literacy, and data visualization and storytelling.
- Data Team Director / Manager: Professionals in this client-facing role oversee advanced analytics projects to outpace their competitors’ analytical accomplishments. For example, they strive to develop better forecasting models and more efficient data collection and preparation processes. Training topics of most interest to data team directors/managers may include data science for managers, fundamentals of data literacy, and data visualization and storytelling.
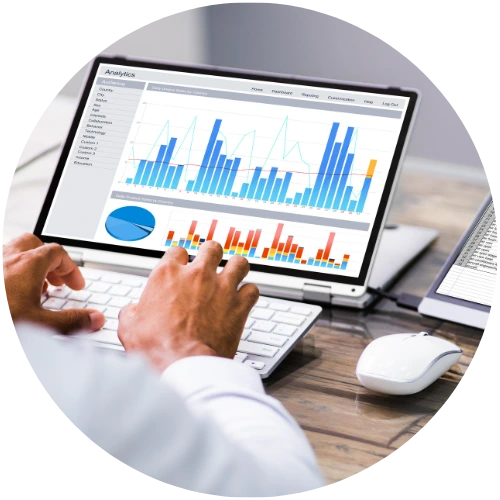
- Data Scientist / Business Analyst: Responsible for corporate data governance, stewardship, quality, transformation, and management, professionals in this role benefit from supplementing and maintaining their knowledge of data science tools and techniques and sharpening the skills they need to communicate effectively about data with professionals in other departments. Relevant training may include storytelling with data, data wrangling in Python, and a range of advanced data science specializations.
Building a Future of Data-Driven Discovery on a Solid Data Management Foundation
Establishing safe and frictionless conduits for data exchange creates boundless opportunities to reduce wasteful redundancies, improve efficiency, and inform better decision-making in healthcare and government. Although organizations and agencies in these fields continue to advance toward a future of seamless data sharing, barriers that have historically inhibited smooth data exchange persist. Therefore, data management at the hands of professionals with essential data science skills will be necessary to ensure this progress continues, delivering improved outcomes for patients and citizens.