Most financial institutions need little persuasion to realize the increasingly critical role of AI/ML technologies in all areas of the industry. However, despite impressive progress embracing these transformative tools, banks and other financial enterprises struggle with their widespread, robust implementation. The heartening news for financial services companies facing these hurdles is that MLOps offers solutions.
The Call for ML in Finance
Financial services companies have long understood that sweeping digitization and advanced data science applications are essential to remaining competitive in today’s business climate. To underscore this point, QuantumBlack AI by McKinsey estimates banking institutions and insurance companies who implement AI/ML solutions ethically and responsibly can potentially see $2 trillion in combined value annually for financial services. In addition, according to an NVIDIA survey, 83% of financial services companies agreed that “AI is important to their company’s success.”
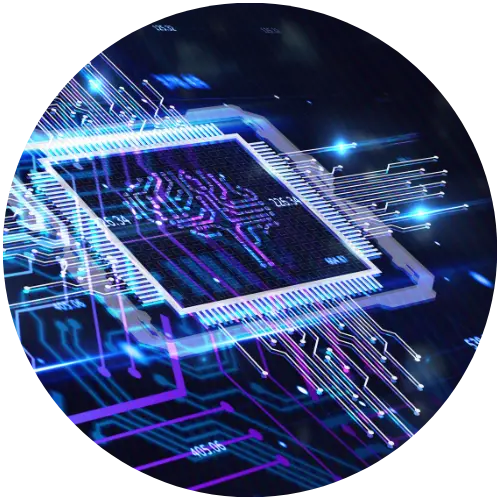
AI/ML technologies offer boundless possibilities for automating processes, improving decision-making, and addressing emerging challenges in the industry. Such challenges include issues related to several current trends that have given rise to a new set of priorities in the financial services industry. For example, the proliferation of data and fintech-driven conveniences have altered customer expectations for their interactions with financial products and services, creating a greater need for personalized digital experiences. In addition, regulations, compliance requirements, and fraud detection are among the top issues financial services must confront while expanding ML programs.
The Challenges to ML Adoption
Although AI/ML technologies offer solutions that can address such demands with speed and reliability, only 13% of financial services companies use AI in most of their processes. In addition, of 750 business leaders surveyed in 2020, only 8% reported that their companies’ ML programs were sophisticated. The common obstacles to fuller ML deployment are costs, data silos, and the need to cultivate trust in AI solutions.
These challenges can stunt progress toward achieving the ML-driven competencies financial institutions envision. As a result, according to a recent Gartner survey, only 54% of AI models developed progress to production. Yet, this is where MLOps can be a differentiating force.
How MLOps Can Accelerate Widespread ML Adoption
Merging DevOps with data science, MLOps enlists the combined talents of such experts as data engineers, data scientists, business analysts, and IT experts to ensure speedy and secure model development, successful deployment, and ongoing performance maintenance. MLOps teams apply their diverse expertise to shaping and monitoring ML models’ performance at each stage, maintaining the project’s focus on business goals and addressing issues related to model risk, reliability, scalability, and transparency.
MLOps teams provide end-to-end oversight for ML projects through these multidisciplinary efforts, enabling swift, scalable innovation while averting common pitfalls. Deloitte AI Institute’s 2021 “State of AI in the Enterprise” survey notes:
Organizations that document and enforce MLOps processes are twice as likely to achieve their goals; nearly two times more likely to report being extremely prepared for risks associated with AI; and nearly two times more confident that they can deploy AI initiatives in a trustworthy way.
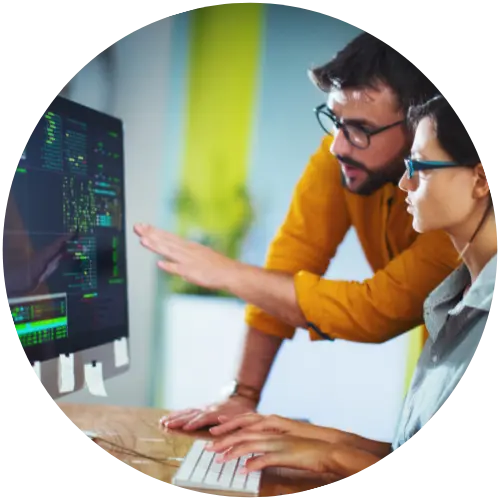
With the rapid evolution of digital technologies and the dynamic stream of data, developing and deploying AI models can present escalated risk for error and regulatory challenges. MLOps methodology addresses these concerns by incorporating data governance and process governance practices into the project workflow to ensure data quality, track potential impact, and ensure the model’s alignment with its target audience and intended outcomes. In addition, MLOps processes can enable teams to embed regulatory, compliance, and ethical requirements into models.
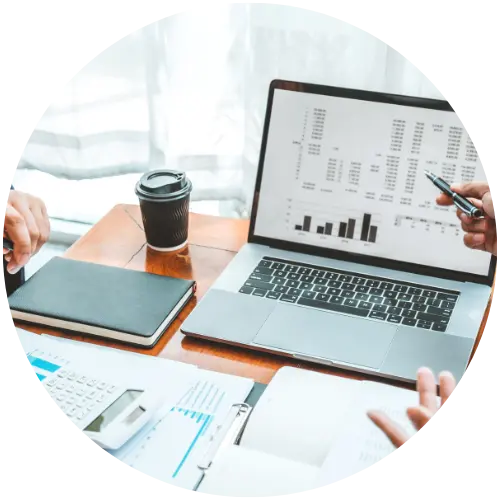
MLOps teams collaboratively manage models throughout their life cycles, seamlessly coordinating to create business value by:
- Automating processes for reduced costs and accelerated workflow.
- Merging siloed data sets to reveal insights relevant across departments.
- Minimizing error.
- Reducing risk.
- Improving audibility and transparency for improved regulatory compliance and explainability.
- Enhancing version control for efficiency and accuracy.
- Increasing model scalability.
The Future of MLOps
MLOps teams help financial institutions implement the AI/ML tools that are increasingly critical in the industry. In addition, as technologies, consumer needs, and business problems continue to change, MLOps methodology will evolve, clearing the path for the financial services industry’s ongoing progress toward a more technologically-powered future.