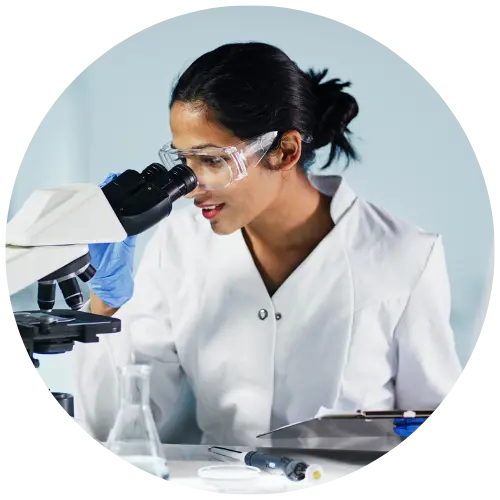
Over the summer months, I’ve discussed the impact of data science technologies in healthcare and life sciences through a series of articles. Part I of the series was an examination of digitization and data usage in these industries. For Part II, I focused on the data science tools and techniques that improve healthcare outcomes. In the following article, the third and final installment of our series, I will explore data science’s contribution to breakthroughs and advances in the life sciences field.
With their rapid adoption of cutting-edge technologies, life sciences organizations are harnessing the power of data science more than ever. Consequently, they’re accelerating the industry’s pace of progress with feats ranging from clinical breakthroughs to improved pharmacovigilance and more impactful commercialization strategies. Life sciences professionals equipped with the tools and training to thrive in this increasingly data-driven field are breaking new ground in all areas.
Harvesting Valuable Insights With Data Literacy
Data science opens a portal to a universe of possibilities for life science advances. However, unlocking this potential requires the knowledge—as well as the technologies—that enable practitioners to draw actionable and reliable conclusions from data. To coax relevant insights from data, interpret them accurately, and translate this information into meaningful guidance for decision-makers, life sciences professionals must hone their data literacy skills.
Many leaders in today’s life sciences industry understand the critical role data literacy plays in driving transformative analytics. According to a recent report, over 50% of pharmaceutical leaders surveyed stated that cultivating data and analytics fluency in their organizations tops their priorities for 2022. Acquiring and maintaining this essential skill set requires ongoing data science training, an effort I believe—given the incredible progress a data-literate workforce can make in all areas of the life sciences industry—is not only worthwhile but vital.
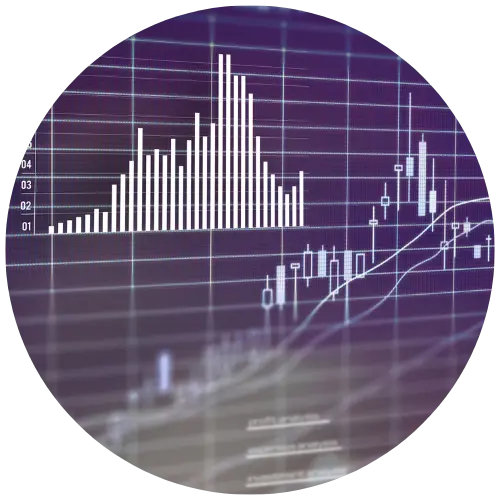
Using Data Science for Better, Safer Products
AI technologies can improve and streamline the quality control process in product development. In addition to automating many of the tasks involved in monitoring product safety, AI can power data-driven analytics capable of issuing advanced warnings of any potentially harmful effects a new drug might cause. For example, ensemble machine learning models can help analysts predict the possible risks present in new drugs by using natural language processing (NLP) to analyze historical records of adverse effects associated with existing drugs.
The industry is also seeing promising developments in deep learning applications for Computer-Aided Drug Design (CADD), which can potentially lead to the use of AI models in place of animal subjects for testing. One study used machine learning technology to predict chemical toxicity to a level of accuracy comparable to that of predictions based on animal testing. Further, AI-enabled agents can use NLP to screen the text of written and spoken consumer inquiries and discern and report issues related to adverse effects. AI and NLP also have the ability to detect and aggregate complaint data for use in models that generate predictions about future safety issues associated with drugs and medical devices on the market.
Leveraging Data Science for Faster Drug Development
Speeding up the lengthy R&D process for faster delivery of new drugs to patients who urgently need them is also a priority for life sciences organizations. Data science technologies are ramping up the pace of the drug development process, which currently takes an average of 10-15 years and $1 billion for each new product that successfully reaches the market. And that is just for the small percentage of drug prospects that reach the approval stage.
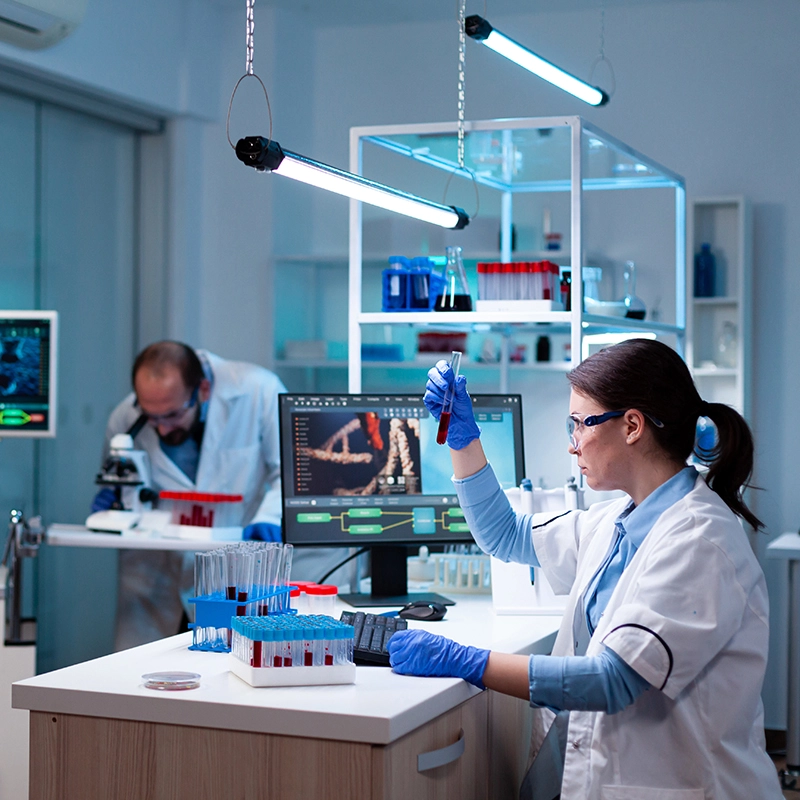
Through automated monitoring of health and safety issues in product manufacturing, data-driven tools can streamline tasks that would otherwise require large investments of human resources and time for supervision and product sampling. In addition, data science offers powerful tools that can quickly identify promising biologics amid a dizzyingly vast field of molecules. This capability reduces the otherwise lengthy analyses such exploration has historically demanded and dramatically decreases the time it takes scientists to determine whether a molecule warrants additional research. Machine learning technology can even aid in the analysis of massive data sets and generate predictions about how a given gene will express under various circumstances, helping researchers target proteins that might play a role in a particular condition.
In skilled hands, data analytics drive decisions about the complexities of clinical trial design, such as subject selection. Data science tools can provide advanced insights into a planned trial’s feasibility, reducing misspent resources, inefficiencies, and delays in the process. Researchers are even investigating possible uses of AI-powered technologies to create external control groups based on data from past clinical trials. This development could potentially eliminate the need for lengthy patient recruitment processes that exclude control subjects from receiving the benefits of trial products.
Optimizing Commercial Effectiveness With Data Science
When approved products approach market readiness, life sciences organizations can leverage data science to improve all phases of the commercialization process. AI-powered tools enable marketers to collect data from a broad range of sources and identify patients and healthcare providers who would likely be most interested in a given product. These data-driven insights can help marketers develop precision strategies for engaging and informing prospective consumers. To track the experience of current customers and monitor problems that may arise with a new product, life sciences companies can employ AI technologies that use NLP to mine structured and unstructured data from digital media for sentiment analysis and tracking of inquiries and complaints. Remarkably, these technologies can also facilitate the onerous processes of regulating promotional compliance issues and even generating copywriting content.
As AI-powered chatbots continue to enjoy increasing applications across industries, life sciences organizations can deploy these virtual agents to gather information about individual consumers’ needs and personalize advertising accordingly. The insights marketers collect from these interactions help them develop targeted campaigns and provide product details to consumers most likely to benefit from the new drug.
Discovering New Insights
AI and ML technologies enable integration of large, multidimensional datasets from numerous sources, facilitating analysis of what would otherwise be overwhelming volumes of complex, heterogeneous data. By mining data from vast repositories and detecting patterns that humans cannot recognize amid oceans of data points, these tools can illuminate significant links.
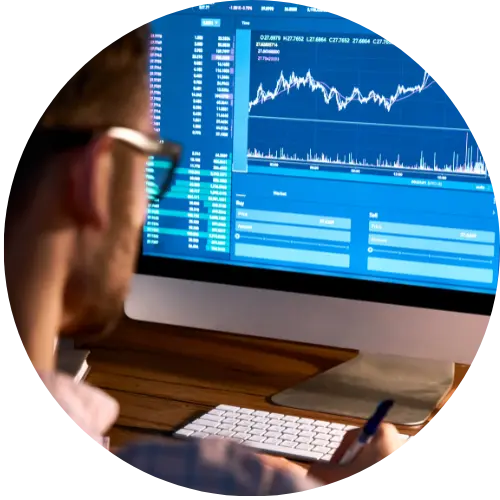
ML is particularly well suited to exploratory analyses of massive datasets, or Knowledge Discovery in Databases (KDD), offering investigative opportunities that differ from analyses that originate with assumptions. Driving revelatory data mining free of preconceived hypotheses, ML offers researchers the capability of discovering previously unimagined relationships between variables.
Uniting disparate data points from widespread sources, ML algorithms can also give researchers fuller views of the complexity of circumstances that correlate to diseases and different health outcomes. AI and ML technologies enable analysis of unstructured and structured data of various types and from different platforms. Knowledge graphs, AI-based tools that map relationships between diverse data points from multiple sources, can aid in link prediction to uncover associations that might otherwise remain hidden in complex and disconnected datasets.
Looking Forward
Despite abundant evidence that life sciences professionals are accelerating breakthroughs and improving operations with data, the industry’s data science revolution is still nascent. Organizations that upskill their teams with training that cultivates data literacy will be at the forefront of data-driven advancements in the field. With the essential data proficiencies, life sciences professionals will be empowered to source, manage, derive, and communicate reliable and relevant information from data and translate it into action.