Data Science Makes a Significant Impact on Clinical Trials
Big Data has transformed how we manage, analyze, and apply data across industries. Healthcare, especially, is a notable area where data analytics and data science can make a significant impact. Currently, data science applications are at the forefront of many innovations in healthcare, particularly in pharmaceutical clinical trials.
Along with the emergence of Artificial Intelligence (AI) and Machine Learning (ML) technologies in the past five years, data science unlocks new possibilities in pharmaceutical research and development. AI/ML tools can churn through massive datasets and provide accurate results in just minutes, uncovering valuable insights that would take many hours for humans to perform. Below are some key benefits that data science can bring to the drug development process:
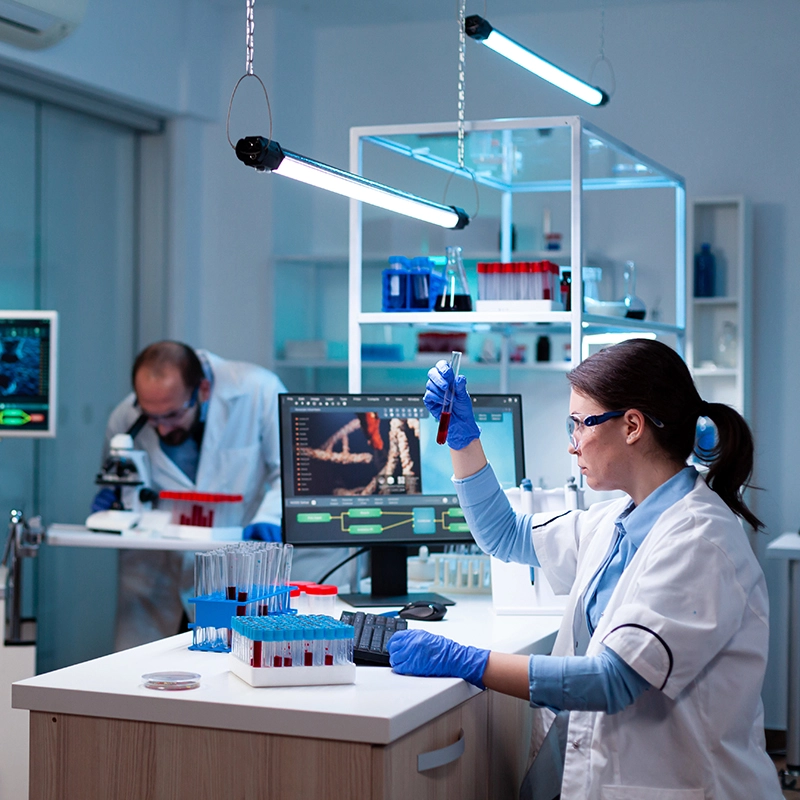
More Accurate Clinical Trials
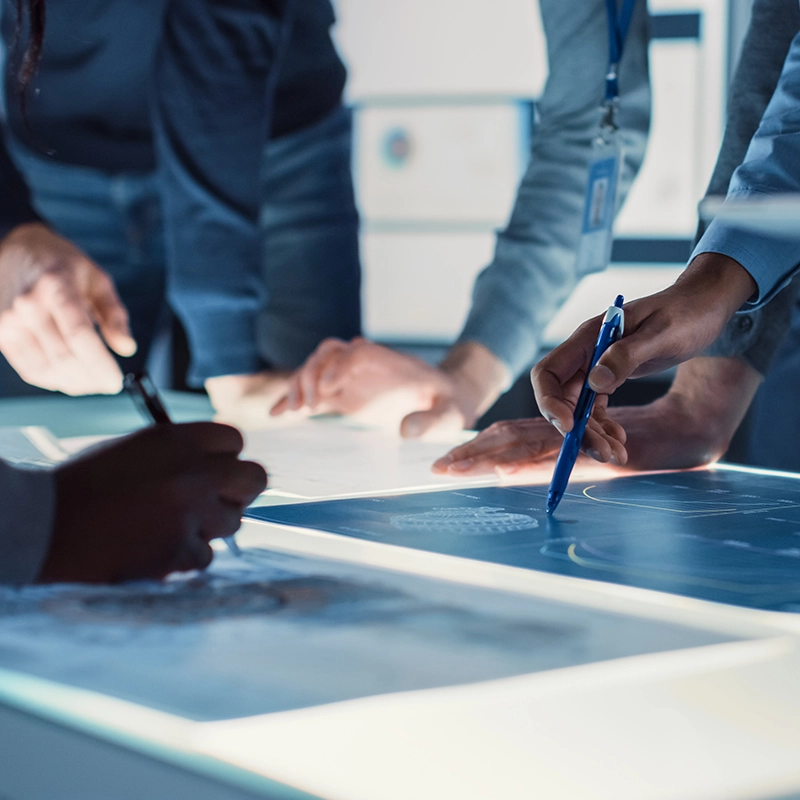
A large pharmaceutical company can have thousands of ongoing clinical trials with millions of datasets. With so many data points, the need for effective data management and data analysis is greater than before. Mismanaged data can lead to costly mistakes that waste precious resources and staff time, or worse, put the entire clinical trial at risk.
Despite these possibilities, many clinical studies still rely on traditional data collection and verification methods, such as counting leftover pills in bottles manually, sending patient medical records via fax, and using patients’ paper diaries to determine their medication adherence. Often, these tasks fall on the patient, who is more likely to forget or make mistakes.
By integrating digital data collection and using advanced technologies like data science, researchers will be able to identify crucial patterns and potential trial complications in real time.
Safer Production of Drugs
Manufacturing a new pharmaceutical drug has historically been a long, arduous process that has relied on manual data processing and collection. However, new applications of machine learning and statistical techniques have led to more streamlined operations that can even help predict the outcomes of randomized clinical trials for new drugs. The result is more accurate and timely estimates of the risks and rewards for all stakeholders involved, including researchers, regulators, and the patients themselves.
In addition to streamlining data collection, scientists can simulate the effects of drugs on the human body by using body proteins and various types of cells and conditions. The resulting drug from that clinical trial is far more likely to be approved by the Food and Drug Administration, as well as cure diverse patient profiles.
With more accurate measures of the risk of drug development, researchers can design improved clinical trials that eliminate costly delays in a market launch. Data science and analytics can also expand the criteria for patient selection. Being able to quickly and accurately sift through factors like individual characteristics, disease status, and genetics helps researchers target patients who match the inclusion and exclusion criteria.
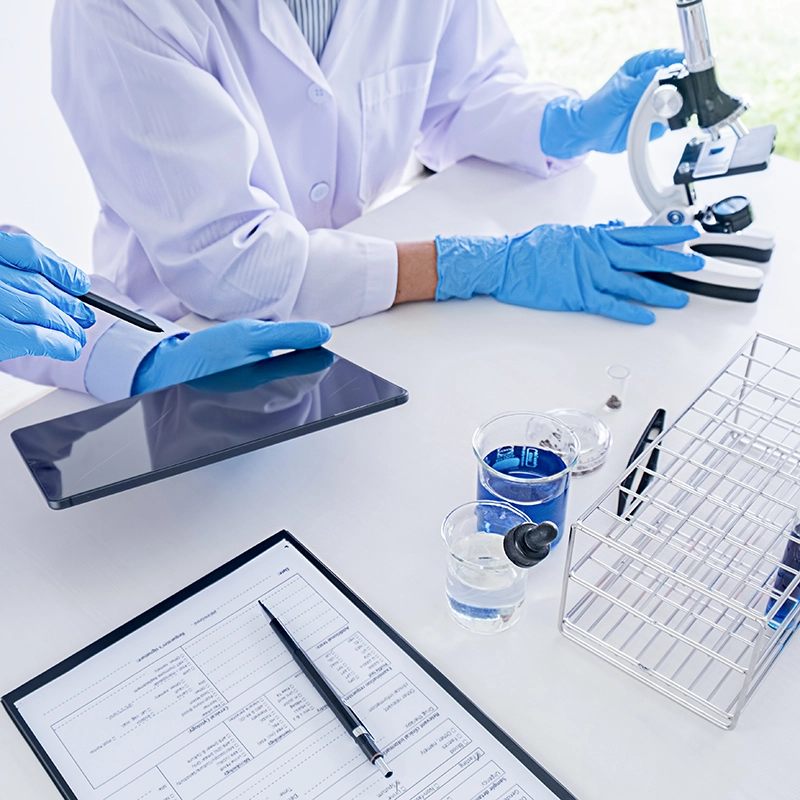
More Efficient Trials
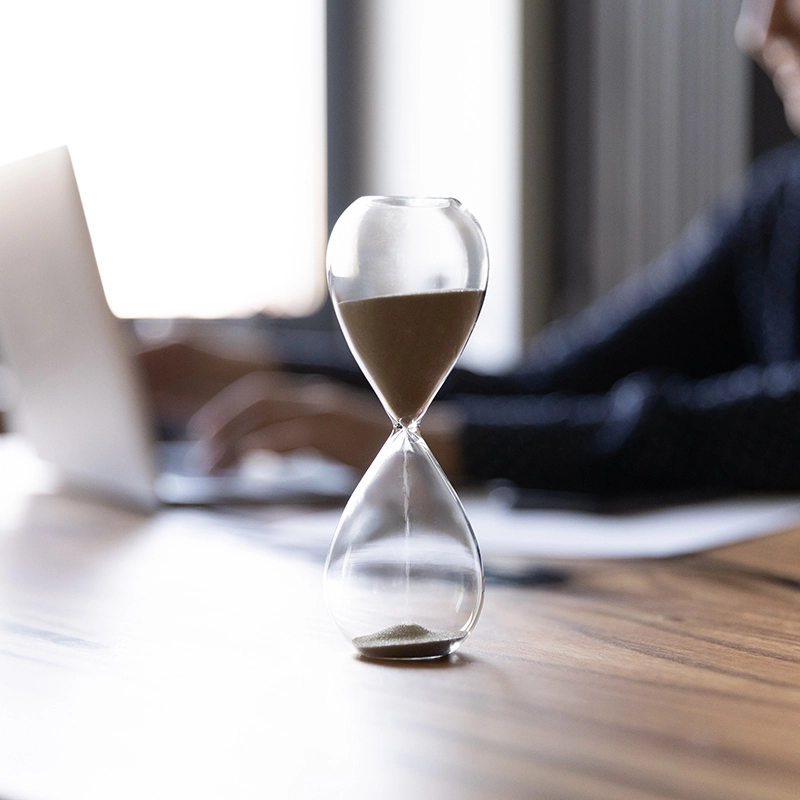
Data analytics not only leads to more informed decision-making during the drug development process but can also improve the efficiency of research and clinical trials. Predictive modeling of drugs and biological processes will become integral to identifying new potential candidate molecules that can be successfully developed into drugs with a high degree of certainty.
By leveraging big data and automation solutions, pharmaceutical companies can respond in real-time to emerging insights from clinical data. They can also be more efficient in their trials by running smaller tests of equivalent power or shorter trial times. These small efficiencies quickly compound and reduce the trial time by a factor of months or even years.
As data continue to pour in from thousands of clinical trials, evolved data strategies will make the drug-development pipeline more efficient. For example, the widespread use of electronic data such as electronic medical records can reduce the likelihood of data errors due to manual or duplicate entry.
New Data Analytics, New Tools
Despite the rise of big data, many organizations still rely on old technologies for data collection and analysis. For years, Microsoft Excel and SAS have been the preferred data analysis tools for professionals in nearly every setting, including clinical research and trials during drug development. While they both have been effective in past research efforts, the acceleration of data collection and processing power has led to a revolution of new flexible tools.
To take advantage of big data analytics, organizations have turned to alternatives such as R programming and Python. R is a free and open-source programming language that offers advanced data analysis functions and capabilities. Compared to Excel, R programming can handle larger data sets and generate more detailed visualizations. Researchers constantly introduce new packages as part of their dissertations, which also creates a community of individuals who keep R on the cutting-edge of scientific research. With clinical trial data, R can streamline complex processes to reduce error and reliably reproduce results.
Python is another programming language that can develop system backends, web pages, or even games. In Python, data scientists can manipulate data like functions in Excel and import data similar to SAS. However, they can also make use of advanced statistics and machine learning capabilities. Python can scale and work with more extensive and multiple datasets. It can also handle large volumes of data at faster processing speeds, which is suited well for data storage of thousands of patient entries and millions of tables. Finally, Python can automate much of the data reports and analyses to save hours of manual work.
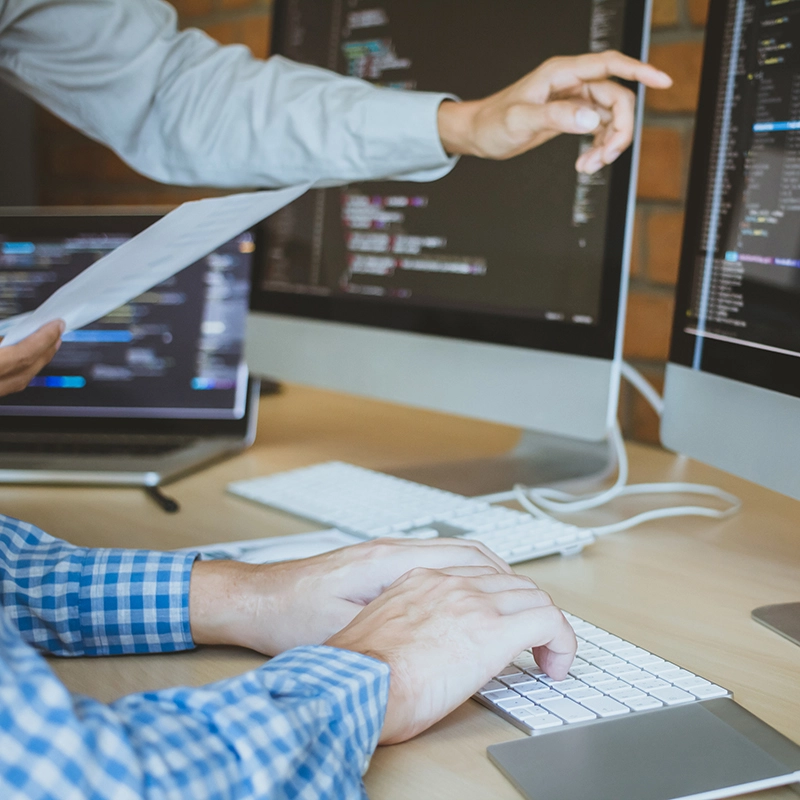
Conclusion
Taking a new pharmaceutical drug to market is a slow and costly process with frequent roadblocks, but big data and data science have reduced risks in drug development. Thanks to improved data strategies and AI/ML solutions, organizations can expedite life-saving drugs through clinical trials in the drug discovery process. The key to unlocking these powerful capabilities is to ensure that your staff understand how to implement algorithms, data collection, storage, validation, and visualization to generate valuable insights.
With professional training, your organization can develop an expert data science team and level up existing employees to suggest data science projects and facilitate collaboration and communication. Data Society will prepare your organization to handle its Big Data and help create competitive advantages during drug development.
Toward a Healthcare Data Revolution
Whitepaper
With more data science applications in healthcare around the globe, it is clear the industry’s transformation has already begun. However, achieving the data maturity required to leverage these capabilities effectively does not come without significant infrastructure, culture, and education challenges.
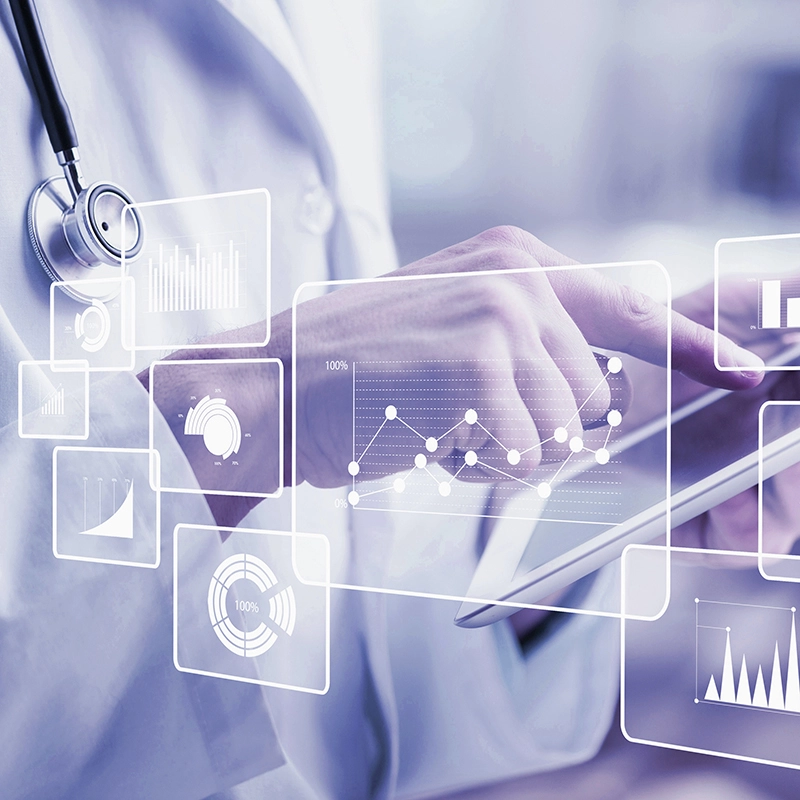
We’ll explore how to start a data science movement that impacts
- Hospital Operations
- Personalized Medicine
- Patient Self-Care
- Public Health